By Rick Kawamura
Social media data continues to grow at astronomical rates. Last year Twitter grew 1,444 percent
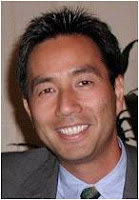
The numbers are absolutely astounding. But is social media data credible? And can tangible business intelligence (BI) be extracted from it? [Disclosure: Kapow Technologies is a sponsor of BriefingsDirect podcasts.]
Reality Buzz, a new social media analysis project powered by web data services technology, was created to answer this very question by examining if real-time analysis of social media conversations can predict the outcome of popular reality television shows like American Idol and Dancing with the Stars. After Reality Buzz collected tens of thousands of tweets, comments, and discussions about contestants on both programs each week and applied sentiment analysis to the data, there was very clear, data driven insight to predict the contestants to be eliminated.
Stepping outside the example of "reality" TV, social media sentiment can be a powerful source of data that arms organizations with real-time intelligence to make more strategic business decisions. Based on experience with Reality Buzz, here are five tips for extracting real value from social media data:
Data trumps conventional wisdomThe growing mass of social media data is definitely a treasure trove of insight to extract intelligence, whether predicting reality show winners or moving your business forward. When done correctly, collecting and analyzing social media sentiment can be a pain-free, powerful tool for real-time feedback, predictive analytics and getting the competitive edge you need to win.
While Malcolm Gladwell, author of Blink: The Power of Thinking Without Thinking, would say otherwise, data-driven business decisions definitely outperform guesswork. Week after week on Dancing with the Stars, the infamous Kate Gosselin held up to 40 percent of all conversations in social media. Unfortunately for Kate, 95 percent of those comments were negative.
Conventional wisdom said that she should pack her bags. Yet the data showeddespite all the negative conversations, she still had more share of positive comments than several other contestants, meaning she was far less likely to be eliminated. Because viewers vote for contestants they’d like to keep on the show, there is a strong correlation to positive sentiment. It wasn’t until the fourth week that Kate’s volume of positive comments died down and she was voted off.
Product managers deal with this dilemma all the time. Tasked with determining the next set of product features to drive greater profitability, they have to manage the CEO’s gut feel while also satisfying the needs of those who have to sell it, both of whom want it better, cheaper and faster. But “better, cheaper, faster” isn’t a great long-term strategy. A great product manager would look to the data to find unmet needs and untapped markets, and social media is a great place to find these hidden nuggets of intelligence.
Timing is critical
Any data over 24 hours old is pretty much worthless for predicting who will be eliminated from a reality TV show. The same holds true in the business world, where it’s imperative for the data to be as close to an event as possible, as this data has the strongest effect on sentiment.Weeks old data may prove costly, resulting in more damage to the brand and revenue.
When launching a new product, for example, companies need to consider sentiment immediately prior to and after the launch. The same applies to a marketing campaign. Say Toyota releases a full page ad in The Wall Street Journal only to get a report on sentiment a few weeks later. Worthless. Companies need to know their customer’s sentiment just before they publish the ad to create the most relevant message, and immediately following to measure its resonance with their audience. Weeks-old data may prove costly, resulting in more damage to the brand and revenue by further demonstrating lack of understanding and responsiveness to frustrated customers.
Don’t be blind to the noise factor
It’s easy to understand trends, changes in momentum, volume of traffic, and ratio of positive to negative sentiment. However there is a lot of noise that can easily skew the data, especially with large, very public shows like American Idol. The bigger the show, product, etc., the more noise. This is most prominent in Twitter, which very often represents the largest source and volume of data. Despite the noise, though, there is valuable information that shouldn’t be ignored. Interestingly, most of the noise resides in neutral sentiment, not positive or negative. These are comments, articles, and reviews about a brand that don’t provide any real opinion.
This is why it’s important to understand how to filter the data to maintain its quality and relevance.
Not all social media sentiment created equal
Companies need to clearly define their goals before analyzing social media data. There are differing degrees of sentiment, and not all translate equally well. Most sentiment analysis tools begin by separating data into positive and negative groups. Yet even within each fan group there are varying degrees of support for contestants. In trying to determine the number of votes for a contestant, consider this data: “I just voted 100 times for Casey” vs. “My top 3 are Lee, Michael and Casey” vs. retweeting a link to a video or article which mentions Casey.Companies also need to consider how to weigh one tweet versus a Facebook comment versus a blog post.
The reality is that not all data is needed or equal in weight. For American Idol, votes are cast for the person you want to keep on the show, so negative sentiment has little correlation to who will be voted off. This requires factoring out negative comments from total sentiment to get the most accurate prediction. Companies also need to consider how to weigh one tweet versus a Facebook comment versus a blog post. Each is just one piece of data, but does each one count equally?
Don’t look at data in a vacuum
Having knowledge of events and circumstances is critical to understanding and extracting intelligence from social media data. In the case of Reality Buzz, it was helpful to watch the performance shows for added context. This process is key for companies to raise other hypotheses to further investigate after they’ve seen the output.
Similarly, some manual data review is also essential to ensure quality and consistency. For example, when using an automated sentiment analysis tool, companies can weigh keywords differently. In addition, automated tools are not yet capable at distinguishing sentiment as functional, emotional or behavioral. So in monitoring social media data, there had to be a huge difference between “I like my new Canon camera” and “I just told my friend to buy the new Canon camera.” While both positive sentiments, the latter should be weighed much more heavily.
Rick Kawamura is Director of Marketing at Kapow Technologies, a leading provider of Web data services. Rick was most recently VP of Marketing at DeNA Global, and previously held strategic and product management roles at Palm and Sun Microsystems. He can be reached at rick.kawamura@kapowtech.com.You may also be interested in:
- Web data services extend business intelligence depth and breadth across social, mobile, web data
- Web data services -- here's why text-based content access and management plays crucial role in a real-time BI
- Web data services provide ease of data access and distribution from variety of sources, destinations
No comments:
Post a Comment